Understanding the Advantages of Flow Sampling: Maximizing Efficiency without Breaking the Bank

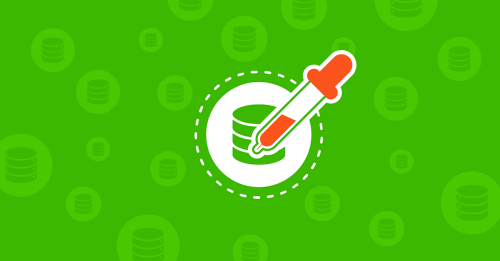
Summary
Does flow sampling reduce the accuracy of our visibility data? In this post, learn why flow sampling provides extremely accurate and reliable results while also reducing the overhead required for network visibility and increase our ability to scale our monitoring footprint.
The whole point of our beloved networks is to deliver applications and services to real people sitting at computers. So, as network engineers, monitoring the performance and efficiency of our networks is a crucial part of our job. Flow data, in particular, is a powerful tool that provides valuable insights into what’s happening in our networks for ongoing monitoring and troubleshooting poor-performing applications.
What is flow data?
Flow data is a type of metadata derived from packets that summarizes the information embedded in a network stream. We use flow data to monitor IP conversations, protocol activity, applications, to see trends, and to identify patterns in traffic. The network devices generate this metadata in the form of flow records sent to flow collectors, usually over the production network.
However, the volume of data that needs to be processed, especially in large networks with high-speed links, can be overwhelming to the network device creating the flow records and the monitoring system. To solve this problem, we can use sampling.
What is flow sampling?
Sampling is a method used to reduce the amount of flow data that needs to be processed by a network device, such as a router or a switch, as well as the monitoring system. As traffic traverses a link, the network device selects a subset of packets to represent the whole, rather than make a copy of every single packet. It then sends this sampled data as a flow record to a flow collector for processing and analysis.
Consider a router in a busy network with very high-speed links. Since the router itself is our de facto monitoring device, we use it both to monitor network traffic as well as for its primary function, to forward packets. The problem is that capturing every single packet and generating many flow records is a massive burden to the local CPU, flow cache, and the network itself, though flow records are lightweight.
So we can configure a sampling rate to capture only a portion of those packets crossing the link and generate a flow record from this sampled data. Based on your needs, you may collect only 1 out of every 1,000 packets, for example, reducing the amount of information you need to process locally and by your monitoring system.
Pros and cons of network flow sampling
The benefits of sampling are pretty straightforward. Your network devices aren’t taxed as heavily, your monitoring system doesn’t have to process as much, and you aren’t adding as much extra traffic to your production network.
This means we can scale our monitoring to a much larger footprint of network devices, servers, containers, clouds, and end-users — a scale that may be nearly impossible otherwise. Sampling allows us to improve our monitoring tools’ performance and scope since we can process large amounts of data much more efficiently.
However, a common argument against sampling is that capturing only a subset of packets gives us incomplete visibility and potentially reduces the accuracy of the results. In other words, sampling costs us visibility and can lead to underrepresentation of the data since there’s less of it collected. This results in an inaccurate picture of the network and our conclusions based on the data.
Accuracy and sampling rate
Though this concern may be valid in certain scenarios, it usually isn’t an issue because of the size of the dataset or, in statistics terms, the population we’re dealing with. Millions and millions of packets traverse a typical router or switch link in a very short period of time. And in statistics, the larger the population, the more accurate the samples will represent the population.
Let’s look at an example.
A sampling rate of 1:1,000 means you’re collecting statistics from one packet out of every 1,000. So, in this case, you’re ignoring the information embedded in those other 999 packets. That’s where the concern arises for some.
We might ask ourselves, “What am I not seeing that could be in those other 999 packets?”
In reality, you’d be capturing a statistically significant sample of a vast dataset when capturing one out of every 1000 packets (a sampling rate of 1:1,000) on a typical 1Gbps link. And that would give you enough information about your network without the fear of overwhelming your routers or monitoring system.
This is because the sampling rate of 1:1000 provides a sufficiently large sample to reflect the entire flow accurately. So if you’re using a sampling rate of 1:1000 and a randomly sampled sampling mode, and if a flow has 100,000 packets and you’re able to sample 100 of them, you’ll have a statistically significant idea of what the other 99,000 packets looked like. In fact, you’ll likely be able to identify the average packet size correctly and even figure out how many bytes were transmitted within a fraction of a percent. This is why most providers employ sampling to determine bandwidth and peering choices.
And therein lies the problem. You have to consider your specific scenario; if your network devices can handle creating and sending a lot of flow records, if you have the available bandwidth to accommodate the additional flow record traffic, and what level of resolution you need in the first place.
Are you looking for the very highest resolution visibility possible? Then you’ll want a sampling rate of 1:1 or pretty close to that, assuming your devices can handle it. If you’re ok with seeing trends, IP conversations, what applications are taking up the most bandwidth, and what endpoints are the chattiest, you can bump that sampling rate to 1:100, 1:1,000, or even 1:10,000.
But is there a sweet spot of sampling enough to be able to scale but not sampling so much that the data becomes useless? Like almost everything in tech, the answer to how much sampling is just right is “it depends.”
Sampling trade-offs
When monitoring a high-speed network like the type used in some banking and finance organizations, keep in mind that individual transactions happen quickly in a short amount of time. Though sampling shouldn’t affect accuracy in most situations, a sampling rate of 1:10,000 could miss vital visibility information for this type of application. So, in this case, you may want a lower sample rate since there’s so much activity happening quickly. This means allocating more resources to your monitoring systems, like CPU and storage, and it means your network devices will have to work a little harder.
On the other hand, a large school district in the United States should be able to get more than enough network visibility information from more sampled flow data. With thousands of students, various devices (including personal devices), and thousands of teachers and staff, a school district would be hard-pressed to handle a low sample rate both in terms of the staff to handle the information, the budget required to process and store the data, and the toll it takes on the network itself.
The inaccuracy we get from sampling is statistically small and generally within acceptable limits for network operators. So, in general, it’s a good practice to start with a low sampling rate and then adjust it as needed based on the type of links being monitored, the volume of data being captured, and your desired level of resolution. It’s also a good idea to monitor your network devices themselves to ensure that the sampling rate is not causing any issues, such as high CPU or memory usage.
As with most things in tech, there are always trade-offs. You adjust your sampling rate according to your specific scenario and understand there may be a slight decrease in accuracy — the cost is a decrease so small that it may be statistically irrelevant.
Even so, this tradeoff is worth it because we can scale our network monitoring to a much greater number and type of devices both on-premises and in the cloud. In the end, sampling costs us a few packets, so we see less. But it allows us to scale our visibility, so we see more.