AI That Matters: Driving Real Outcomes in Network Operations

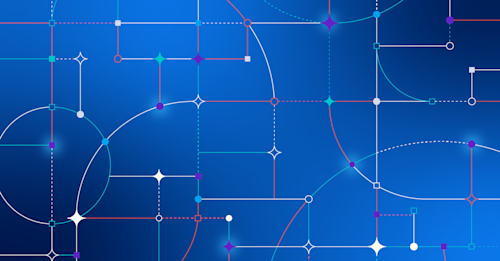
Summary
AI can be a transformative tool in network operations — but only when it’s tied to clear, measurable outcomes. Rather than chasing hype, IT and NetOps teams should focus on solving specific operational challenges like reducing MTTR, cutting costs, and stabilizing infrastructure. AI has real potential when strategically applied, and when aligned with business goals, it becomes a powerful ally in modern network operations.
There’s a buzz going on in the world of network operations, and it revolves around one big question: is all this talk about AI just hype, or can it deliver tangible benefits?
It’s essential to remember that AI is a means to an end, not the end itself. Success with AI begins by defining specific, measurable outcomes with operational impact.
Of course, there’s certainly a “cool factor” with AI right now, which is understandable. After all, AI brings all kinds of advanced capabilities, from classical rules-based engines to machine learning to the latest large language models. However, we must focus on precisely what we want to achieve in NetOps. We can’t know if and how AI is the right approach until we identify our operational pain points, such as unstable infrastructure or rising cloud costs.
The biggest goals for many NetOps teams involve stabilizing infrastructure, shrinking MTTR, and stretching their budget further by reducing tickets and identifying network design and operations efficiencies. Once those metrics are on the table, we can shift from “let’s do AI” to “what will help us reach these targets most effectively?”
This kind of clarity is critical because AI itself should never be considered the ultimate goal. It’s just one technology among many, and sometimes it’s not even the most cost-effective or practical solution.
For example, in the data center, there may be a rash of incidents tied to old optical transceivers from a hardware refresh. We can absolutely spin up a full-blown AI pipeline, with a fancy model that ingests streams of real-time telemetry from switches and optics. This pipeline could run logistic regression or even deep learning models to predict which components are most likely to fail.
Sounds incredible — and it can be. But if the cost of architecting, building, and maintaining such a solution outweighs simply swapping out faulty transceivers, then the glitz of AI won’t pay off in the long run. Sure, the AI approach might impress upper management for a while, but it’s unlikely to hold up under real-world scrutiny if it isn’t sustainable or justifiable.
On the other hand, there are situations where AI truly can outperform more conventional strategies. Remember that the networks we manage today generate an enormous amount of telemetry of different types, from various sources, and often in near-real time. Add to that the body of text in the form of device configs, tickets, KB articles, and so on, and we have an almost insurmountable mass of data to mine through to manage our infrastructure.
Network observability allowed us to unify much of this body of data and apply telemetry pipelines and workflows to bring us out of the dark age of traditional monitoring. In the same way, using modern AI workflows to network observability can make the interrogation of data seamless and provide deeper insight and analysis than ever before.
The definitive guide to running a healthy, secure, high-performance network
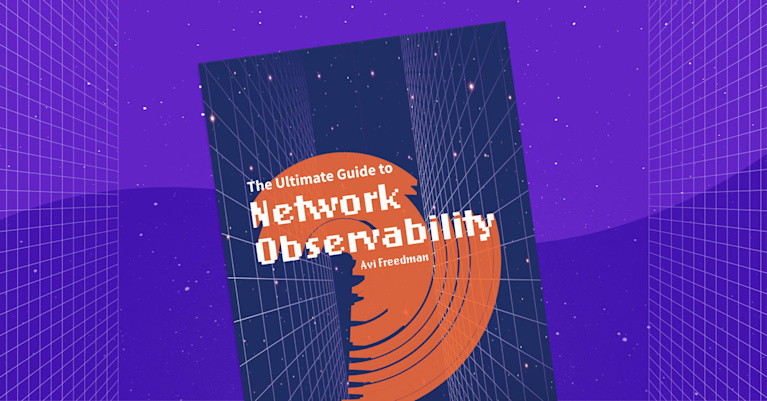
An AI-driven system can help pinpoint the root cause of repeated failures, highlight clusters of troublesome configurations, or even predict emerging problems. That’s where AI steps into the limelight with real, quantifiable impact: fewer repeated incidents, a shorter time spent diagnosing new ones, and an overall reduction in user frustration.
Of course, the key is ensuring that AI never exists for its own sake. Many IT teams have, at one time or another, fallen prey to the lure of the latest shiny technology. This isn’t new in our industry. A revolutionary solution bursts onto the scene, and everyone clamors to implement it without a specific plan. When this happens, it’s not unusual to see PoCs go on forever and projects fail because it doesn’t actually solve an operational problem. AI is just as prone to that fate as anything else. If there isn’t a robust framework for measuring success (like decreased downtime or faster incident resolution), or if the path to achieving those improvements with AI is too convoluted, the project will fizzle out.
As Eric Siegel explains in his book, The AI Playbook, architecture is strategic, AI is tactical. In other words, our overall architecture is a strategic matter involving business outcomes, operational workflows, KPIs, budget constraints, etc. AI, on the other hand, is the tactical tool to achieve those outcomes.
Strategy involves big-picture thinking about how data flows through the organization, how various systems integrate and speak to each other, and how operational workflows can be refined to deliver real results. Only after that strategic foundation is in place is it time to decide whether AI is the correct tactic. This approach protects organizations from racing down a rabbit hole of over-engineered solutions or going down the build path when buying a pre-built and supported solution would have been a much better route.
Organizations today face constant pressure to innovate, but innovation must serve operational and business aims to be sustainable. AI can absolutely be a powerful force in network operations — from predictive analytics to advanced LLM-driven insights. However, this only holds if the technology is anchored in real objectives that matter to the organization. Think of it as bridging the gap between ambition and action. AI might still grab headlines and generate excitement, but the winning strategy is always to use it where it does the most good. In other words, AI’s role is to serve the outcomes that define real success, whether that’s increased uptime, reduced costs, or a more streamlined and rewarding experience for IT users everywhere.
Network operations teams can move forward confidently by focusing on outcomes first, knowing they’ve aligned AI with clear goals. The bottom line is that operational improvement should catalyze every technology decision, and AI is no exception. When deployed with the right objectives, AI can improve network operations by solving real, day-to-day operational problems.