Data-Driven AI: The Key to Network Observability

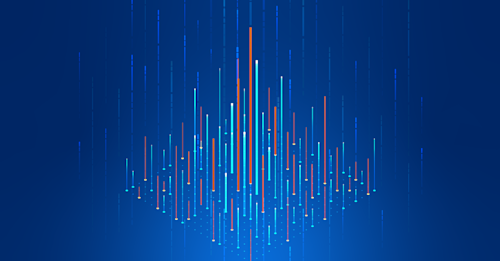
Summary
AI has the power to optimize network performance, detect anomalies, and improve capacity planning, but its effectiveness depends on high-quality data. Without a strong data foundation, AI remains a theoretical tool rather than delivering real operational value.
AI has captured the imagination of many industries, promising transformative insights and automation. In the realm of network operations, AI has the potential to revolutionize performance optimization, anomaly detection, capacity planning, and the ability to glean meaningful insight from network telemetry. However, realizing these benefits isn’t as simple as flipping a switch. AI thrives on high-quality data, and the reality is that most organizations underestimate the complexities involved in achieving this foundational requirement.
Without sufficient high-quality data, AI becomes an experiment or a theoretical exercise rather than a practical tool. Understanding the data challenges of AI is essential for network admins, cloud engineers, and IT managers to deploy solutions that deliver real value.
The importance of data to AI
An AI system will only be as good as the data we feed it. Poor quality or insufficient data leads to inaccurate predictions, unreliable results, and ineffective automation. To be of any real value, AI systems need vast amounts of data to learn patterns and make reliable predictions. The nature of networking, in particular, poses some challenges to an effective AI implementation.
First, networks are complex, dynamic systems with diverse telemetry sources. Network telemetry includes a range of data, such as flows, metrics, routing tables, contextual metadata, etc. This results in two problems: dealing with a massive volume of data and wildly different data types.
Second, this raw data is often riddled with noise, inconsistencies, errors, and missing values. Cleaning this data—removing outliers, filling missing values, and ensuring consistency—is critical for AI to work effectively. In fact, data processing and preparation are often the bulk of the activity involved in implementing an AI solution.
Next, AI insights need to be based on up-to-date information. Stale data can result in irrelevant decisions, especially in dynamic network environments. Handling real-time telemetry data in an AI workflow is a challenge for even skilled data scientists and engineers, so careful attention must be given to how telemetry data is ingested.
Lastly, data must be efficiently stored, processed, and accessible to AI models in real or near-real times. A poorly designed database can prevent efficient queries and produce inaccurate results. High latency between an AI system and relevant databases can slow a workflow and adversely affect network operations.
Addressing these requirements is not trivial in the least. It requires a sophisticated infrastructure and approach to data management. On top of this are the common challenges network operations teams already face, including data silos, volume overload, and a fragmented ecosystem.
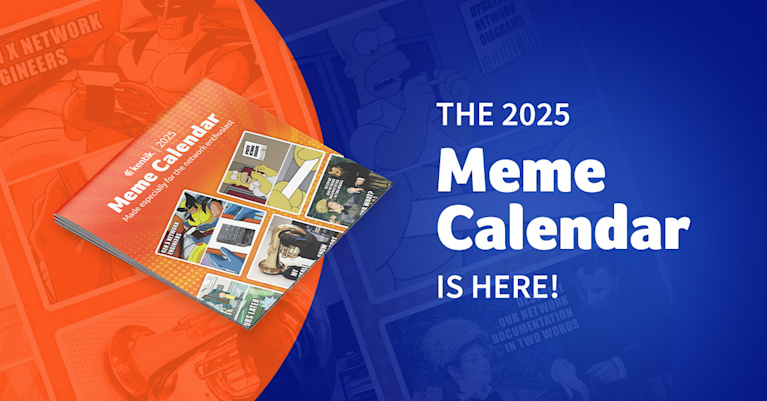
How Kentik addresses the data challenge
Kentik’s platform is purpose-built to solve the data challenges that hinder effective AI deployment in network operations. By focusing on the foundational elements of data ingestion, storage, cleaning, and processing, Kentik enables AI to deliver actionable insights with accuracy and relevance.
Kentik’s approach to data can be broken down into several components.
Kentik ingests a significant variety of network telemetry data, including flow records (NetFlow, sFlow, IPFIX), SNMP metrics, streaming telemetry, cloud flow logs, BGP routing tables, and enriched metadata. This breadth ensures that AI models have access to the diverse data required to understand the full context of network behavior.
The platform was designed from the beginning to handle the massive scale of modern networks. Kentik’s advanced storage architecture retains detailed telemetry data over time, enabling longitudinal analysis and access to rich historical data.
A showstopper for many organizations interested in implementing an AI solution is data processing. Kentik automatically processes raw telemetry to eliminate noise and inconsistencies. This includes deduplication, normalization across data types, and enrichment with additional contexts, such as geolocation and device-level and organization-level metadata.
Kentik’s real-time analytics engine processes incoming data as it’s generated, providing the low-latency insights required for day-to-day network operations. This ensures that AI-driven alerts and recommendations are always based on the latest network information.
Because Kentik already ingests, cleans, and processes vast volumes of network telemetry, it’s perfectly positioned to support AI models. Rather than starting from scratch, organizations can leverage Kentik’s pre-built infrastructure and automated intelligence functionality to accelerate their own AI initiatives.
Kentik Journeys
Journeys provides an AI-augmented user experience built on this foundation of data. Since Kentik already ingests, processes, and updates vast amounts of network telemetry data, engineers and IT managers using Journeys can gain insights from data more efficiently and perform advanced analyses to achieve a meaningful understanding without architecting, building, and maintaining sophisticated data repositories.
By solving the most complex parts of the data challenge, Kentik enables network operators to focus on AI’s outcomes rather than the intricacies of data preparation. For network engineers and IT professionals, this means that Kentik doesn’t just make AI possible; it makes AI practical and effective for network operations.