Leveraging Big Data for Continuous Improvement

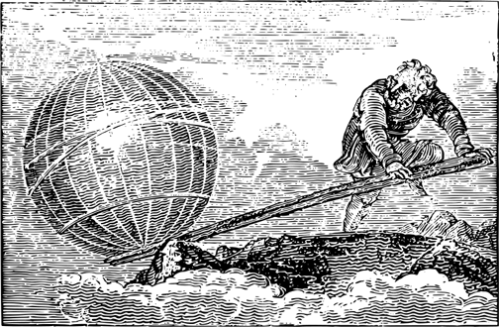
Summary
Intelligent use of network management data can enable virtually any company to transform itself into a successful digital business. In our third post in this series, we look at areas where traditional network data management approaches are falling short, and we consider how a Big Data platform that provides real-time answers to ad-hoc queries can empower IT organizations and drive continuous improvement in both business and IT operations.
The Digital Business Benefits of Data-driven Network Management
The advantages of applying a Big Data approach to business operations have been widely discussed, including in the article “When Big Data Goes Lean” from McKinsey & Company, which identifies the impact that Big Data is having on manufacturing. “The application of larger data sets, faster computational power, and more advanced analytic techniques,” the authors say, “is spurring progress on a range of lean-management priorities. Sophisticated modeling can help to identify waste, for example, thus empowering workers and opening up new frontiers where lean problem solving can support continuous improvement. Powerful data-driven analytics also can help to solve previously unsolvable (and even unknown) problems that undermine efficiency in complex manufacturing environments: hidden bottlenecks, operational rigidities, and areas of excessive variability. Similarly, the power of data to support improvement efforts in related areas, such as quality and production planning, is growing as companies get better at storing, sharing, integrating, and understanding their data more quickly and easily.”
Proactive Analytics for Network Security
The potential benefits from what McKinsey describes as “larger data sets, faster computational power, and more advanced analytic techniques” aren’t limited to manufacturing. Network security is another example of an area with lots of potential for data-driven improvement. Until the notorious 2014 breach of Target’s credit card database, security was widely perceived as being just an IT issue. That changed when Target’s profits dropped by almost 50% and the company fired not just their CIO but also their CEO. The belated realization that security was a core issue has since been reinforced on an ongoing basis by reports such as the IBM X-Force Threat Intelligence Report 2016, which pointed out that by 2019 cybercrime will become a 2.1 trillion dollar problem.
Network security is an area with lots of potential for data-driven improvement.
Because the potential financial impact is now indisputable, security has become a priority concern for CIOs, CEOs, and boards. Particularly unsettling is the fact that organizations may not recognize when security has been compromised, meaning that months can elapse between the start of a breach and its resolution. The extent to which organizations have unknown security problems was highlighted in the 2015 Cost of Data Breach Study. The study found that malicious attacks can take an average of 256 days to identify while data breaches caused by human error take an average of 158 days. The study also pointed out that the the ultimate cost of containing a given security issue is directly linked to how long it takes to identify it.
With the stakes so high, it’s clear that network security is an area that can benefit greatly from enabling experts to implement continuous improvement and to solve challenging or hidden problems. Network operations and network security groups regularly see anomalous traffic patterns and are faced with the question: “Is this traffic shift indicative of an organic change, a misconfiguration, or some form of security breach?” That seemingly simple question is nearly impossible to answer adequately within the confines of traditional network management tools. As discussed earlier in this series, legacy network analytics systems store only relatively small amounts of management data and use siloed GUIs. Without a comprehensive, unified view of network data — enabled by a distributed Big Data architecture to handle traffic at scale — there’s no effective way to share the detailed traffic insights that would allow various network teams to work together to address common problems.
Troubleshooting and Preventing Application Issues
When applications are key to running the business, ensuring performance is critical.
Another priority issue for a company’s IT organization and business leaders alike is to ensure acceptable application performance. This is particularly critical if the application is key to running the business. As pointed out in the 2015 Application and Services Delivery Handbook, the management task that organizations say is most important for them to get better at in the near term is rapidly identifying the source of degraded application performance.
In part, troubleshooting is a high priority because poor performance in customer-facing applications typically leads to reduced revenue. But another reason is that troubleshooting is getting more challenging. Network operators must now manage new application delivery models that increasingly rely on public cloud services and include mobile workers, virtualized infrastructure, and new application types such as cloud-native. The growing complexity and distributed nature of applications means that there are more angles from which network operations needs to look at management data to rapidly solve performance or availability issues. Traditional network management based on summary-only views doesn’t allow access to the details needed to support that sort of analysis.
Time for Big Data Network Management
As network complexity grows it’s increasingly evident that only a Big Data solution can cope. But as pointed out in our second post, not all Big Data-based analytics platforms are created equal. Systems developed for business intelligence (BI) analytics rely on batch processing and may require hours to run a single query. To be effective for network operations and security, however, an analytics solution must enable users to get a response to an individual query in seconds. Timely answers enable network operations and network security personnel to combine their technical expertise and institutional knowledge of their organization’s infrastructure with insight gained from rapid, detailed, iterative querying. This combination is the formula for solving application and availability problems faster. Ideally, these teams will use these deep analytical capabilities to proactively examine anomalies that point to sub-optimal conditions and then fix those issues before they impact users or compromise security.
Instead of continuing with traditional approaches, IT organizations should empower workers with comprehensive, real-time data-driven analytics.
Einstein is often credited with saying that insanity can be defined as doing the same thing over and over again while expecting a different result. The traditional approach to combatting security incidents has led growing financial impact of cybercrime, which is expected to be a 2.1 trillion dollar problem by 2019. The traditional approach to troubleshooting degraded application performance has led to it being consistently identified as the area in which network organizations most need near term improvement. While continuing with traditional approaches and expecting to get notably better at security or troubleshooting may not be insane, it does seem foolhardy. Instead, IT organizations should adopt a Big Data network management solution that empowers workers with comprehensive, real-time data-driven analytics. By leveraging that power with their own expertise, network teams will be able to seek continuous improvement and to address problems that they haven’t previously been able to solve or even see.