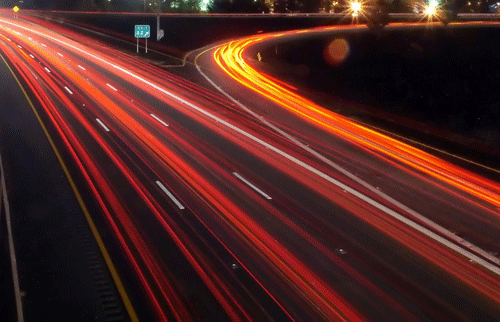
Summary
SDN holds lots of promise, but it’s practical applications have so far been limited to discrete use cases like customer provisioning or service scaling. The long-term goal is true dynamic control, but that requires comprehensive traffic intelligence in real time at full scale. As our customers are discovering, Kentik Detect’s traffic visibility, anomaly detection, and extensive APIs make it an ideal source for actionable traffic data that can drive network automation.
Traffic Intelligence is the Key to Effective Network Automation
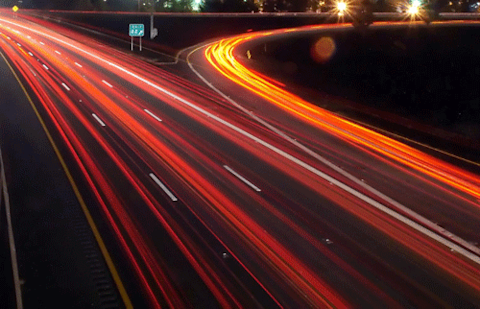
Reading the tech press, one might understandably conclude that software defined networks (SDN) are “eating the world” (to borrow from Marc Andreesen). Some of this excitement is justified, because SDN holds lots of promise for improving the way that we build, scale, and operate network infrastructure. As our traffic grows and network loads become increasingly dynamic, it’s difficult to keep pace using manual provisioning. So we have SDN/overlay technologies like VXLAN to simplify provisioning of connectivity between VMs running on diverse physical hosts, and NFV in service-provider networks to simplify deployment of customer services. To date though, practical, deployable SDN technology has mostly been limited to these niches. Meanwhile the hype of SDN goes far beyond automating and simplifying network provisioning.
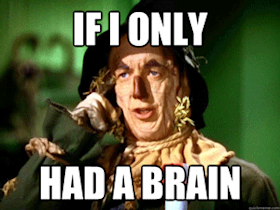
Where Da Brain?
SDN advocates often tout the promise of things like “self-driving networks” that execute automatic responses to adverse conditions such as attacks and congestion. What’s been delivered so far, though, focuses primarily on network programmability. SDN APIs, interfaces, and orchestration typically enable operators to respond to discrete external events, like customer provisioning or service scaling, by reconfiguring the network from a central point of control. Getting from that to a truly self-driving network will require a feedback loop in which brain-like components consume massive amounts of information about network traffic and dynamically re-program the network based on metrics and analytics.
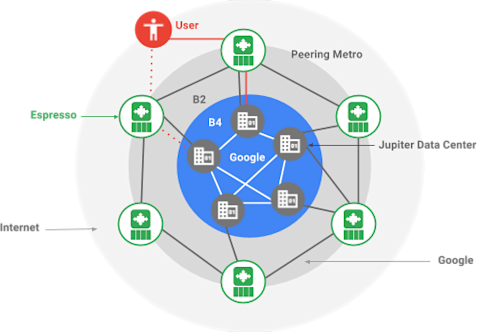
By and large, the SDN components available in commercially consumable solutions haven’t yet achieved that level of intelligence, though hypergiants like Google and Facebook are making progress with in-house projects. Google recently published some details on their Espresso peering edge architecture which uses metrics from their various apps to dynamically choose the datacenter/location from which an end user will be served, optimizing for loss, latency, and other factors to deliver the best user experience.
In a similar fashion, Facebook recently published a blog describing their Express Backbone that provides internal connectivity between datacenters. A major component of the system is the ability to continuously generate a matrix of site-to-site traffic loads based on metrics (sFlow) collected from the network. The system then dynamically provisions an MPLS LSP topology to meet the observed loads while optimizing for various traffic classes (e.g. latency sensitive vs. insensitive).
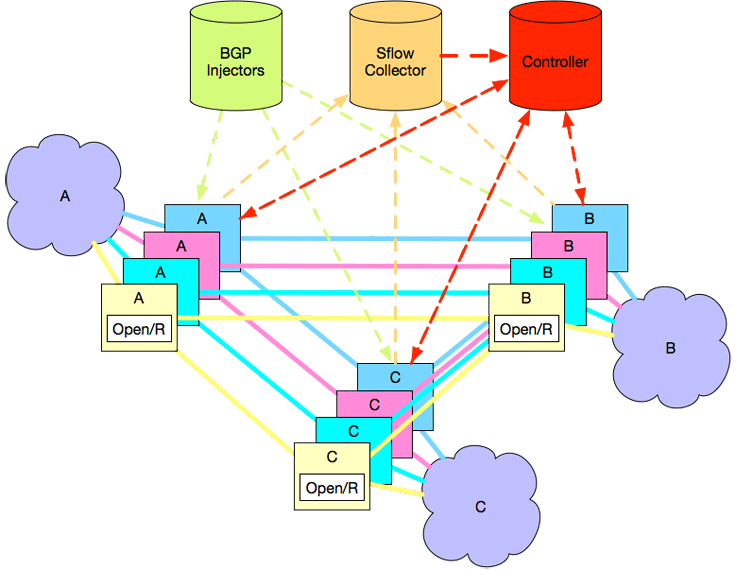
Like the SDN hype in the press — and the collateral from network hardware vendors — Facebook glosses over the component on their diagram that is labeled “Sflow Collector,” which is what actually provides inputs to the controller. Google’s Espresso blog post says “we leverage our large-scale computing infrastructure and signals from the application itself…” which gets a little closer to describing the scope of the task. Collecting network traffic metadata at scale and transforming it into network control inputs, all in real-time, is a significant engineering challenge. What if you don’t have an army of in-house software engineers to craft a purpose-built, real-time big data platform?
Automation-ready Traffic Intelligence
Kentik was essentially founded as a response to the above question. Our API-enabled SaaS, Kentik Detect, is a single, central service for collecting, processing, and analyzing flow data, and it produces outputs that arm network operators with visibility, forensics, automated detection, and — increasingly — automated control. Using the built-in DDoS detection feature set, for example, you can automatically orchestrate a number of different network actions. RTBH route injection via BGP will drop malicious traffic at the network edge. And API integration with partners like Radware and A10 enables automated triggering of DDoS scrubbing hardware when Kentik Detect identifies traffic that is outside of either absolute parameters or baseline norms.
Kentik Detect also enables customers such as Pandora to optimize user experience while cutting IP transit costs. Pandora’s feedback loop relies on Kentik APIs to observe traffic volumes and network conditions. Translated into actionable intelligence, the data from Kentik Detect helps determine (similar to Google’s Espresso SDN) the servers from which individual end-users are served. And it also guides automated manipulation of routing to control the transit involved in reaching those users. Pandora’s senior director of network operations and engineering, James Kelty, discusses the company’s use of Kentik Detect in this video.
Watch this space for further posts on how Kentik Detect’s big data-powered network traffic intelligence helps drive automation in our customers’ networks. We’re excited to help our customers achieve the full promise of SDN and be part of the move toward the “self-driving network.” If you’re looking to build more self-driving capabilities into your network, Kentik Detect is the network traffic intelligence component needed to provide actionable network data to your automation subsystems. You can learn more about Kentik Detect on our our product pages, or let us show it to you by scheduling a demo. Or try it out directly by starting a free trial today.